
[ad_1]
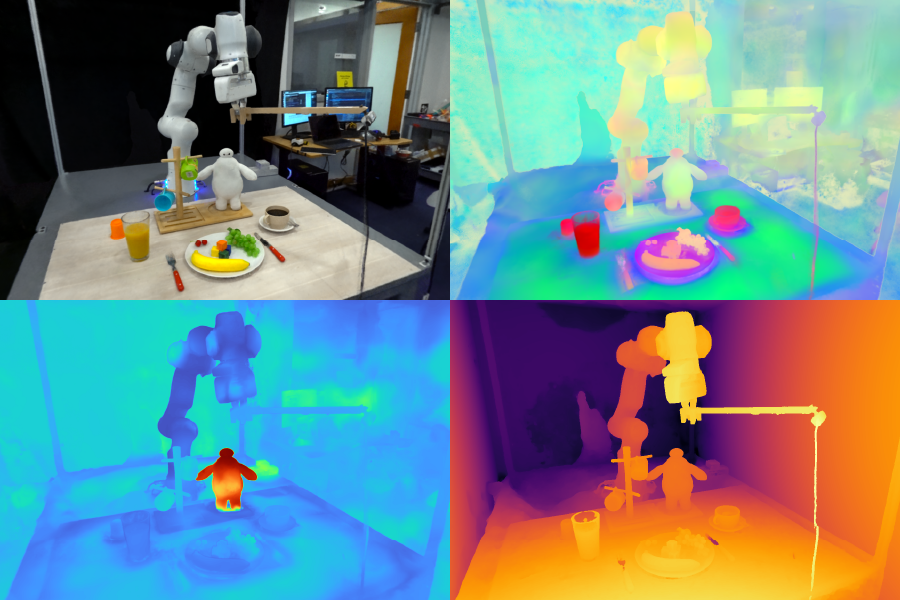
Characteristic Fields for Robotic Manipulation (F3RM) permits robots to interpret open-ended textual content prompts utilizing pure language, serving to the machines manipulate unfamiliar objects. The system’s 3D characteristic fields may very well be useful in environments that comprise hundreds of objects, comparable to warehouses. Photos courtesy of the researchers.
By Alex Shipps | MIT CSAIL
Think about you’re visiting a pal overseas, and also you look inside their fridge to see what would make for a fantastic breakfast. Lots of the gadgets initially seem overseas to you, with each encased in unfamiliar packaging and containers. Regardless of these visible distinctions, you start to know what each is used for and decide them up as wanted.
Impressed by people’ skill to deal with unfamiliar objects, a gaggle from MIT’s Pc Science and Synthetic Intelligence Laboratory (CSAIL) designed Characteristic Fields for Robotic Manipulation (F3RM), a system that blends 2D photographs with basis mannequin options into 3D scenes to assist robots determine and grasp close by gadgets. F3RM can interpret open-ended language prompts from people, making the tactic useful in real-world environments that comprise hundreds of objects, like warehouses and households.
F3RM presents robots the power to interpret open-ended textual content prompts utilizing pure language, serving to the machines manipulate objects. Because of this, the machines can perceive less-specific requests from people and nonetheless full the specified job. For instance, if a person asks the robotic to “decide up a tall mug,” the robotic can find and seize the merchandise that most closely fits that description.
“Making robots that may truly generalize in the actual world is extremely laborious,” says Ge Yang, postdoc on the Nationwide Science Basis AI Institute for Synthetic Intelligence and Basic Interactions and MIT CSAIL. “We actually wish to work out how to try this, so with this challenge, we attempt to push for an aggressive degree of generalization, from simply three or 4 objects to something we discover in MIT’s Stata Middle. We wished to discover ways to make robots as versatile as ourselves, since we are able to grasp and place objects regardless that we’ve by no means seen them earlier than.”
Studying “what’s the place by trying”
The tactic might help robots with selecting gadgets in massive achievement facilities with inevitable muddle and unpredictability. In these warehouses, robots are sometimes given an outline of the stock that they’re required to determine. The robots should match the textual content supplied to an object, no matter variations in packaging, in order that clients’ orders are shipped accurately.
For instance, the achievement facilities of main on-line retailers can comprise hundreds of thousands of things, a lot of which a robotic can have by no means encountered earlier than. To function at such a scale, robots want to know the geometry and semantics of various gadgets, with some being in tight areas. With F3RM’s superior spatial and semantic notion talents, a robotic might grow to be more practical at finding an object, inserting it in a bin, after which sending it alongside for packaging. Finally, this may assist manufacturing facility staff ship clients’ orders extra effectively.
“One factor that always surprises folks with F3RM is that the identical system additionally works on a room and constructing scale, and can be utilized to construct simulation environments for robotic studying and huge maps,” says Yang. “However earlier than we scale up this work additional, we wish to first make this method work actually quick. This manner, we are able to use this kind of illustration for extra dynamic robotic management duties, hopefully in real-time, in order that robots that deal with extra dynamic duties can use it for notion.”
The MIT workforce notes that F3RM’s skill to know completely different scenes might make it helpful in city and family environments. For instance, the method might assist customized robots determine and decide up particular gadgets. The system aids robots in greedy their environment — each bodily and perceptively.
“Visible notion was outlined by David Marr as the issue of figuring out ‘what’s the place by trying,’” says senior writer Phillip Isola, MIT affiliate professor {of electrical} engineering and pc science and CSAIL principal investigator. “Current basis fashions have gotten actually good at figuring out what they’re taking a look at; they will acknowledge hundreds of object classes and supply detailed textual content descriptions of photographs. On the similar time, radiance fields have gotten actually good at representing the place stuff is in a scene. The mixture of those two approaches can create a illustration of what’s the place in 3D, and what our work exhibits is that this mixture is very helpful for robotic duties, which require manipulating objects in 3D.”
Making a “digital twin”
F3RM begins to know its environment by taking photos on a selfie stick. The mounted digicam snaps 50 photographs at completely different poses, enabling it to construct a neural radiance discipline (NeRF), a deep studying methodology that takes 2D photographs to assemble a 3D scene. This collage of RGB pictures creates a “digital twin” of its environment within the type of a 360-degree illustration of what’s close by.
Along with a extremely detailed neural radiance discipline, F3RM additionally builds a characteristic discipline to enhance geometry with semantic info. The system makes use of CLIP, a imaginative and prescient basis mannequin educated on lots of of hundreds of thousands of photographs to effectively study visible ideas. By reconstructing the 2D CLIP options for the photographs taken by the selfie stick, F3RM successfully lifts the 2D options right into a 3D illustration.
Conserving issues open-ended
After receiving a couple of demonstrations, the robotic applies what it is aware of about geometry and semantics to know objects it has by no means encountered earlier than. As soon as a person submits a textual content question, the robotic searches by way of the area of doable grasps to determine these probably to achieve selecting up the thing requested by the person. Every potential possibility is scored primarily based on its relevance to the immediate, similarity to the demonstrations the robotic has been educated on, and if it causes any collisions. The very best-scored grasp is then chosen and executed.
To reveal the system’s skill to interpret open-ended requests from people, the researchers prompted the robotic to select up Baymax, a personality from Disney’s “Huge Hero 6.” Whereas F3RM had by no means been immediately educated to select up a toy of the cartoon superhero, the robotic used its spatial consciousness and vision-language options from the inspiration fashions to resolve which object to know and decide it up.
F3RM additionally permits customers to specify which object they need the robotic to deal with at completely different ranges of linguistic element. For instance, if there’s a metallic mug and a glass mug, the person can ask the robotic for the “glass mug.” If the bot sees two glass mugs and one among them is stuffed with espresso and the opposite with juice, the person can ask for the “glass mug with espresso.” The inspiration mannequin options embedded throughout the characteristic discipline allow this degree of open-ended understanding.
“If I confirmed an individual decide up a mug by the lip, they may simply switch that data to select up objects with comparable geometries comparable to bowls, measuring beakers, and even rolls of tape. For robots, attaining this degree of adaptability has been fairly difficult,” says MIT PhD pupil, CSAIL affiliate, and co-lead writer William Shen. “F3RM combines geometric understanding with semantics from basis fashions educated on internet-scale information to allow this degree of aggressive generalization from only a small variety of demonstrations.”
Shen and Yang wrote the paper below the supervision of Isola, with MIT professor and CSAIL principal investigator Leslie Pack Kaelbling and undergraduate college students Alan Yu and Jansen Wong as co-authors. The workforce was supported, partially, by Amazon.com Companies, the Nationwide Science Basis, the Air Pressure Workplace of Scientific Analysis, the Workplace of Naval Analysis’s Multidisciplinary College Initiative, the Military Analysis Workplace, the MIT-IBM Watson Lab, and the MIT Quest for Intelligence. Their work will likely be introduced on the 2023 Convention on Robotic Studying.
MIT Information
[ad_2]