
[ad_1]
ChatGPT and different deep generative fashions are proving to be uncanny mimics. These AI supermodels can churn out poems, end symphonies, and create new movies and pictures by mechanically studying from hundreds of thousands of examples of earlier works. These enormously highly effective and versatile instruments excel at producing new content material that resembles all the things they’ve seen earlier than.
However as MIT engineers say in a brand new research, similarity isn’t sufficient if you wish to really innovate in engineering duties.
“Deep generative fashions (DGMs) are very promising, but in addition inherently flawed,” says research writer Lyle Regenwetter, a mechanical engineering graduate pupil at MIT. “The target of those fashions is to imitate a dataset. However as engineers and designers, we frequently don’t wish to create a design that’s already on the market.”
He and his colleagues make the case that if mechanical engineers need assist from AI to generate novel concepts and designs, they must first refocus these fashions past “statistical similarity.”
“The efficiency of numerous these fashions is explicitly tied to how statistically comparable a generated pattern is to what the mannequin has already seen,” says co-author Faez Ahmed, assistant professor of mechanical engineering at MIT. “However in design, being totally different could possibly be vital if you wish to innovate.”
Of their research, Ahmed and Regenwetter reveal the pitfalls of deep generative fashions when they’re tasked with fixing engineering design issues. In a case research of bicycle body design, the crew exhibits that these fashions find yourself producing new frames that mimic earlier designs however falter on engineering efficiency and necessities.
When the researchers introduced the identical bicycle body downside to DGMs that they particularly designed with engineering-focused goals, quite than solely statistical similarity, these fashions produced extra modern, higher-performing frames.
The crew’s outcomes present that similarity-focused AI fashions don’t fairly translate when utilized to engineering issues. However, because the researchers additionally spotlight of their research, with some cautious planning of task-appropriate metrics, AI fashions could possibly be an efficient design “co-pilot.”
“That is about how AI can assist engineers be higher and quicker at creating modern merchandise,” Ahmed says. “To try this, now we have to first perceive the necessities. That is one step in that route.”
The crew’s new research appeared just lately on-line, and will probably be within the December print version of the journal Laptop Aided Design. The analysis is a collaboration between pc scientists at MIT-IBM Watson AI Lab and mechanical engineers in MIT’s DeCoDe Lab. The research’s co-authors embrace Akash Srivastava and Dan Gutreund on the MIT-IBM Watson AI Lab.
Framing an issue
As Ahmed and Regenwetter write, DGMs are “highly effective learners, boasting unparalleled potential” to course of big quantities of information. DGM is a broad time period for any machine-learning mannequin that’s skilled to be taught distribution of information after which use that to generate new, statistically comparable content material. The enormously widespread ChatGPT is one sort of deep generative mannequin referred to as a big language mannequin, or LLM, which includes pure language processing capabilities into the mannequin to allow the app to generate life like imagery and speech in response to conversational queries. Different widespread fashions for picture era embrace DALL-E and Secure Diffusion.
Due to their potential to be taught from knowledge and generate life like samples, DGMs have been more and more utilized in a number of engineering domains. Designers have used deep generative fashions to draft new plane frames, metamaterial designs, and optimum geometries for bridges and automobiles. However for essentially the most half, the fashions have mimicked present designs, with out enhancing the efficiency on present designs.
“Designers who’re working with DGMs are type of lacking this cherry on high, which is adjusting the mannequin’s coaching goal to concentrate on the design necessities,” Regenwetter says. “So, individuals find yourself producing designs which are similar to the dataset.”
Within the new research, he outlines the principle pitfalls in making use of DGMs to engineering duties, and exhibits that the elemental goal of normal DGMs doesn’t take into consideration particular design necessities. As an instance this, the crew invokes a easy case of bicycle body design and demonstrates that issues can crop up as early because the preliminary studying part. As a mannequin learns from hundreds of present bike frames of assorted dimensions and shapes, it would contemplate two frames of comparable dimensions to have comparable efficiency, when in actual fact a small disconnect in a single body — too small to register as a major distinction in statistical similarity metrics — makes the body a lot weaker than the opposite, visually comparable body.
Past “vanilla”
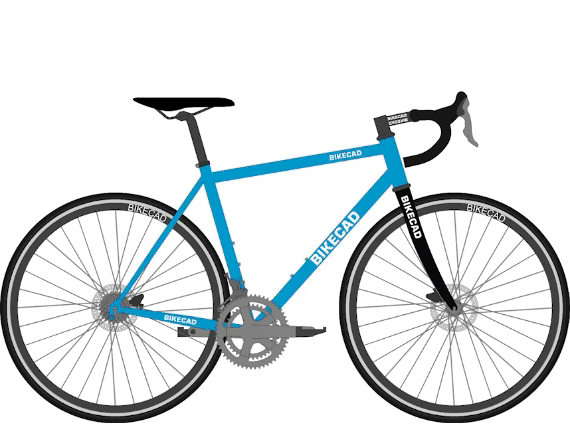
Credit score: Courtesy of the researchers
The researchers carried the bicycle instance ahead to see what designs a DGM would really generate after having discovered from present designs. They first examined a traditional “vanilla” generative adversarial community, or GAN — a mannequin that has extensively been utilized in picture and textual content synthesis, and is tuned merely to generate statistically comparable content material. They skilled the mannequin on a dataset of hundreds of bicycle frames, together with commercially manufactured designs and fewer standard, one-off frames designed by hobbyists.
As soon as the mannequin discovered from the information, the researchers requested it to generate a whole lot of recent bike frames. The mannequin produced life like designs that resembled present frames. However not one of the designs confirmed important enchancment in efficiency, and a few have been even a bit inferior, with heavier, much less structurally sound frames.
The crew then carried out the identical check with two different DGMs that have been particularly designed for engineering duties. The primary mannequin is one which Ahmed beforehand developed to generate high-performing airfoil designs. He constructed this mannequin to prioritize statistical similarity in addition to purposeful efficiency. When utilized to the bike body job, this mannequin generated life like designs that additionally have been lighter and stronger than present designs. Nevertheless it additionally produced bodily “invalid” frames, with parts that didn’t fairly match or overlapped in bodily unattainable methods.
“We noticed designs that have been considerably higher than the dataset, but in addition designs that have been geometrically incompatible as a result of the mannequin wasn’t centered on assembly design constraints,” Regenwetter says.
The final mannequin the crew examined was one which Regenwetter constructed to generate new geometric constructions. This mannequin was designed with the identical priorities because the earlier fashions, with the added ingredient of design constraints, and prioritizing bodily viable frames, for example, with no disconnections or overlapping bars. This final mannequin produced the highest-performing designs, that have been additionally bodily possible.
“We discovered that when a mannequin goes past statistical similarity, it could possibly provide you with designs which are higher than those which are already on the market,” Ahmed says. “It’s a proof of what AI can do, whether it is explicitly skilled on a design job.”
As an example, if DGMs could be constructed with different priorities, similar to efficiency, design constraints, and novelty, Ahmed foresees “quite a few engineering fields, similar to molecular design and civil infrastructure, would significantly profit. By shedding mild on the potential pitfalls of relying solely on statistical similarity, we hope to encourage new pathways and techniques in generative AI purposes outdoors multimedia.”
[ad_2]