
[ad_1]
Abstract: Researchers developed groundbreaking deepfake detection algorithms designed to attenuate racial and gender biases. These algorithms, each demographic-aware and demographic-agnostic, intention to steadiness accuracy throughout totally different teams whereas sustaining and even enhancing general detection charges.
The analysis highlights the necessity for equity in AI instruments and addresses the problem of biased coaching information. The examine, supported by DARPA, demonstrates a major step in the direction of equitable AI options within the realm of deepfake detection.
Key Info:
- The brand new algorithms cut back disparities in deepfake detection accuracy throughout totally different races and genders.
- The demographic-aware technique minimizes errors on much less represented teams, whereas the demographic-agnostic method identifies options unrelated to race or gender.
- Testing confirmed that these strategies not solely improved equity metrics but additionally elevated general detection accuracy in some circumstances.
Supply: College at Buffalo
The picture spoke for itself.
College at Buffalo pc scientist and deepfake professional Siwei Lyu created a photograph collage out of the a whole bunch of faces that his detection algorithms had incorrectly labeled as faux — and the brand new composition clearly had a predominantly darker pores and skin tone.
“A detection algorithm’s accuracy ought to be statistically unbiased from components like race,” Lyu says, “however clearly many present algorithms, together with our personal, inherit a bias.”
Lyu, PhD, co-director of the UB Middle for Data Integrity, and his workforce have now developed what they imagine are the first-ever deepfake detection algorithms particularly designed to be much less biased.
Their two machine studying strategies — one which makes algorithms conscious of demographics and one which leaves them blind to them — decreased disparities in accuracy throughout races and genders, whereas, in some circumstances, nonetheless enhancing general accuracy.
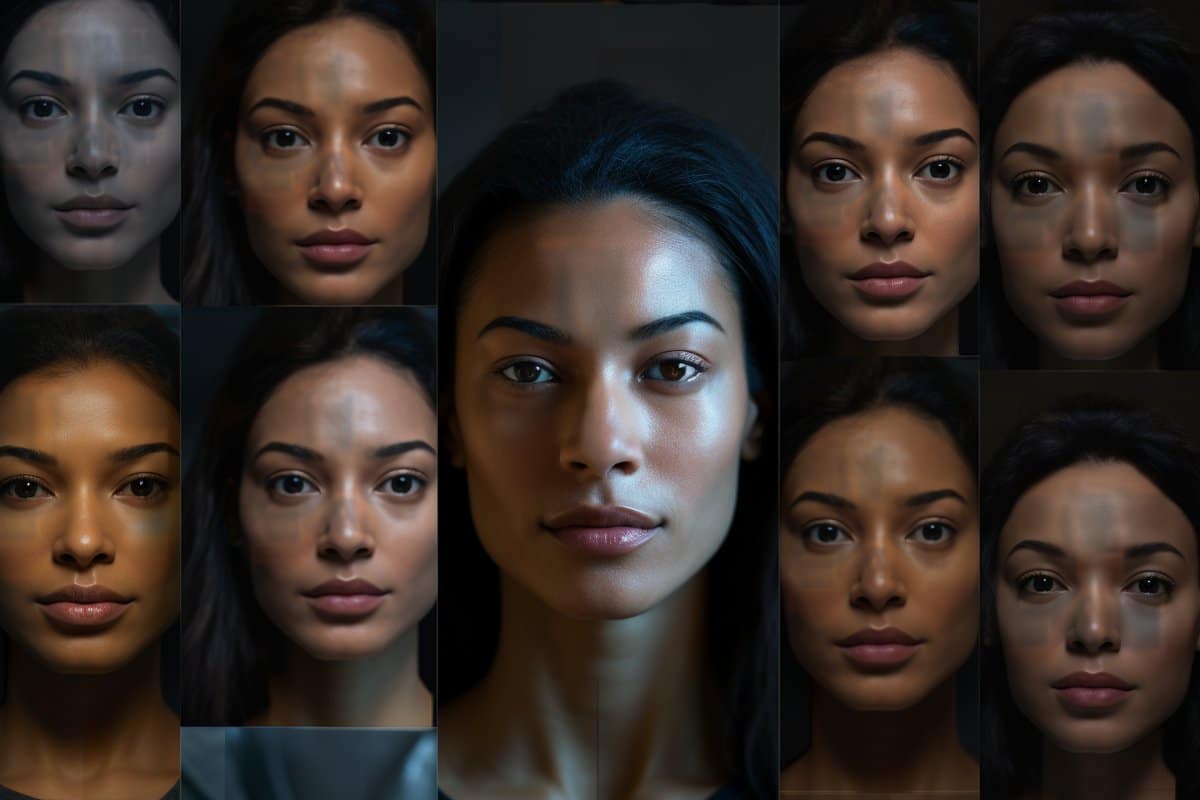
The analysis was offered on the Winter Convention on Purposes of Laptop Imaginative and prescient (WACV), held Jan. 4-8, and was supported partly by the U.S. Protection Superior Analysis Initiatives Company (DARPA).
Lyu, the examine’s senior creator, collaborated together with his former scholar, Shu Hu, PhD, now an assistant professor of pc and knowledge know-how at Indiana College-Purdue College Indianapolis, in addition to George Chen, PhD, assistant professor of knowledge techniques at Carnegie Mellon College. Different contributors embrace Yan Ju, a PhD scholar in Lyu’s Media Forensic Lab at UB, and postdoctoral researcher Shan Jia.
Ju, the examine’s first creator, says detection instruments are sometimes much less scrutinized than the bogus intelligence instruments they maintain in verify, however that doesn’t imply they don’t should be held accountable, too.
“Deepfakes have been so disruptive to society that the analysis neighborhood was in a rush to discover a resolution,” she says, “however regardless that these algorithms had been made for a superb trigger, we nonetheless want to concentrate on their collateral penalties.”
Demographic conscious vs. demographic agnostic
Latest research have discovered massive disparities in deepfake detection algorithms’ error charges — as much as a ten.7% distinction in a single examine — amongst totally different races. Specifically, it’s been proven that some are higher at guessing the authenticity of lighter-skinned topics than darker-skinned ones.
This can lead to sure teams being extra liable to having their actual picture pegged as a faux, or maybe much more damaging, a doctored picture of them pegged as actual.
The issue isn’t essentially the algorithms themselves, however the information they’ve been educated on. Center-aged white males are sometimes overly represented in such photograph and video datasets, so the algorithms are higher at analyzing them than they’re underrepresented teams, says Lyu, SUNY Empire Professor within the UB Division of Laptop Science and Engineering, inside the Faculty of Engineering and Utilized Sciences.
“Say one demographic group has 10,000 samples within the dataset and the opposite solely has 100. The algorithm will sacrifice accuracy on the smaller group with a view to reduce errors on the bigger group,” he provides. “So it reduces general errors, however on the expense of the smaller group.”
Whereas different research have tried to make databases extra demographically balanced — a time-consuming course of — Lyu says his workforce’s examine is the primary try to truly enhance the equity of the algorithms themselves.
To clarify their technique, Lyu makes use of an analogy of a instructor being evaluated by scholar check scores.
“If a instructor has 80 college students do properly and 20 college students do poorly, they’ll nonetheless find yourself with a reasonably good common,” he says. “So as an alternative we wish to give a weighted common to the scholars across the center, forcing them to focus extra on everybody as an alternative of the dominating group.”
First, their demographic-aware technique equipped algorithms with datasets that labeled topics’ gender — male or feminine — and race — white, Black, Asian or different — and instructed it to attenuate errors on the much less represented teams.
“We’re primarily telling the algorithms that we care about general efficiency, however we additionally wish to assure that the efficiency of each group meets sure thresholds, or not less than is barely a lot beneath the general efficiency,” Lyu says.
Nonetheless, datasets sometimes aren’t labeled for race and gender. Thus, the workforce’s demographic-agnostic technique classifies deepfake movies not primarily based on the themes’ demographics — however on options within the video not instantly seen to the human eye.
“Possibly a bunch of movies within the dataset corresponds to a selected demographic group or perhaps it corresponds with another function of the video, however we don’t want demographic info to determine them,” Lyu says. “This fashion, we don’t have to handpick which teams ought to be emphasised. It’s all automated primarily based on which teams make up that center slice of information.”
Bettering equity — and accuracy
The workforce examined their strategies utilizing the favored FaceForensic++ dataset and state-of-the-art Xception detection algorithm. This improved the entire algorithm’s equity metrics, equivalent to equal false constructive charge amongst races, with the demographic-aware technique performing better of all.
Most significantly, Lyu says, their strategies really elevated the general detection accuracy of the algorithm — from 91.49% to as excessive as 94.17%.
Nonetheless, when utilizing the Xception algorithm with totally different datasets and the FF+ dataset with totally different algorithms, the strategies — whereas nonetheless enhancing most equity metrics — barely decreased general detection accuracy.
“There is usually a small tradeoff between efficiency and equity, however we will assure that the efficiency degradation is proscribed,” Lyu says. “After all, the basic resolution to the bias drawback is enhancing the standard of the datasets, however for now, we should always incorporate equity into the algorithms themselves.”
About this deepfake and AI analysis information
Writer: Tom Dinki
Supply: College at Buffalo
Contact: Tom Dinki – College at Buffalo
Picture: The picture is credited to Neuroscience Information
Unique Analysis: The findings had been offered on the Winter Convention on Purposes of Laptop Imaginative and prescient
[ad_2]