
[ad_1]
Within the realm of conventional synthetic intelligence (AI) and the rising generative AI revolution, some truisms stay, significantly “rubbish in, rubbish out.” Actually, this holds extra reality than ever however must be prolonged even additional to incorporate monitoring the rubbish out and in too – that is the function of governance.
As organizations race to combine, and develop AI into their operational workflows, there’s rising consciousness that the standard of information feeding these algorithms is simply as essential because the algorithms themselves.
For big language use instances, this additionally means the info impacts the generated response. The extra modern knowledge that may increase a basis mannequin, the higher the response. For instance, present LLMs don’t perceive present financial circumstances or bleeding-edge AI analysis. Consequently, a LLM is unable to supply modern context and related info. The sustained want for “old style” AI and the rising advantages of generative AI elevate the function of information high quality and governance, making each indispensable components in its profitable utility.
DataRobot’s AI philosophy, constructed on years of predictive AI experience, expands correct governance and analysis layers to all AI workflows, together with generative AI.
Knowledge Integrity: The Basis of Correct Fashions
DataRobot gives knowledge high quality checks and huge language mannequin comparisons.
All AI, each predictive and generative, is a type of sample recognition. AI fashions study patterns from knowledge; therefore, the lineage, integrity, accuracy, and reliability of information are paramount. If the info is flawed as a result of inconsistencies, missingness, duplications, or errors, the AI mannequin’s predictions and analyses might be off-mark. Excessive knowledge high quality ensures that the AI fashions are well-trained and make dependable, correct predictions or generate acceptable, logical responses. With out this, an AI utility can do extra hurt than good, with inaccurate predictions, poor high quality suggestions and, in excessive instances, result in misinformed choices and methods.
Regulatory Compliance and Moral Issues
DataRobot’s automated compliance documentation captures knowledge traits, and mannequin conduct, serving to mannequin threat administration personnel effectively standardize reporting necessities.
Knowledge governance isn’t just an operational concern but additionally a authorized and moral one. With legal guidelines just like the Basic Knowledge Safety Regulation (GDPR) in Europe and the California Shopper Privateness Act (CCPA) within the U.S., organizations are required to deal with knowledge fastidiously. Correct knowledge governance protocols make it simpler to adjust to these rules, decreasing the chance of penalties and reputational injury. Moreover, moral AI requires that knowledge is sourced and processed in a fashion that’s simply and unbiased. Governance constructions and rules-based entry controls assist be sure that knowledge ethics are upheld, as they regulate who can entry and deal with the info to keep away from potential unethical purposes.
For big language fashions and generative AI extra principally, the possession and doable copyright infringement of works utilized in coaching knowledge is being debated amongst coverage makers. Thus, it’s an vital and evolving house worthy of any knowledge chief’s consideration.
Traceability and Accountability
DataRobot’s workflow approvals and deployment studies guarantee auditability and accountability for any AI deployment.
As AI purposes are more and more utilized in essential decision-making processes, with the ability to hint how choices are made turns into vital. Knowledge governance gives a framework for traceability, guaranteeing every knowledge level’s origins, transformations, and makes use of are well-documented. This creates a clear setting the place accountability is obvious and the rationale behind AI-driven choices may be simply defined.
That is significantly essential in sectors like healthcare and finance, the place decision-making has vital implications. The power for a company to audit AI choices publish factum is essential in these regulated and impactful industries. Nonetheless many organizations have poor knowledge possession and oversight, with knowledge transformations and ETL pipelines held captive in knowledge science notebooks with restricted shareability and documentation.
Scalability and Future-Proofing
DataRobot’s AI Platform is the one know-how able to constructing, governing, and working predictive, and generative AI for fashions constructed inside and out of doors of DataRobot, giving organizations the last word flexibility.
As organizations develop, so does the quantity and complexity of their knowledge. Sturdy governance frameworks permit for scalability by guaranteeing that new knowledge integrates seamlessly with present knowledge swimming pools. This ensures that AI fashions stay correct and helpful as they evolve and adapt to new knowledge. Furthermore, a robust concentrate on knowledge high quality ensures that your AI methods are future-proof, able to incorporating new sorts and sources of information as know-how advances. Few organizations have multi-modal modeling in manufacturing and fewer nonetheless make the most of each generative and predictive AI in the identical workflow. The absence of an adaptive knowledge coverage framework, regarding what’s acceptable knowledge use, knowledge supply, and knowledge kind reduces the possibilities of a company being unable to extract worth from many sources comparable to comparable to utilizing textual content summarization inside a predictive modeling workflow or including giant language mannequin context to a predictive worth.
Aggressive Benefit
DataRobot’s sturdy integrations and interoperability with any knowledge supply together with knowledge warehouses and databases like Snowflake or DataBricks ensures you may construct AI irrespective of the place your knowledge lies.
Within the aggressive panorama, the businesses that extract probably the most worth from their AI investments would be the ones that succeed. Excessive-quality knowledge is a potent aggressive benefit, enabling extra correct insights, higher buyer experiences, and simpler decision-making. Actually, many organizations excel solely as a result of their knowledge is superior to that of their trade friends. Having distinctive knowledge assortment and governance can result in lowered prices, elevated income, and, in some instances, solely new markets. Governance constructions assist keep this high quality benefit, making it defensible in opposition to rivals and a degree of differentiation.
Lowering Prices and Dangers
DataRobot’s AI Platform permits you to evaluate the tradeoff in easier fashions, normally less expensive, to correct responses so organizations can choose the optimum predictive or generative AI for the duty.
Dangerous knowledge is expensive. Based on IBM, poor knowledge high quality prices the U.S. economic system round $3.1 trillion yearly. Errors should be corrected, unhealthy choices revisited, and deceptive insights clarified—all of which devour useful time and assets. And that’s simply conventional, predictiveAI! As organizations rely extra closely on generative AI responses, unhealthy knowledge can yield hallucinations that appear credible but are factually incorrect. The outlandish generative AI response shouldn’t preserve enterprise leaders awake at night time, their workers will determine it simply. The believable but inaccurate generative AI response is the problematic one. A governance framework minimizes these dangers by establishing protocols for knowledge high quality, validation, and utilization to assist mitigate pricey AI errors.
In Conclusion
The applying of AI isn’t just a technical endeavor however an organizational one, requiring an interdisciplinary strategy with a deep understanding of information high quality and governance. With AI fashions taking part in an more and more integral function in decision-making and operations, the integrity of the info fueling all AI fashions turns into a essential concern. Organizations that acknowledge the significance of information high quality and governance are higher positioned to develop AI purposes which can be correct, dependable, moral, and, finally, extra useful in reaching enterprise objectives.
Concerning the writer
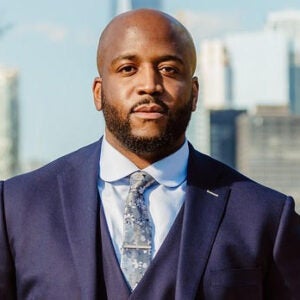
As Government Director and Head of Enterprise Intelligence and Superior Analytics at Mindshare, Ikechi helps shoppers to leverage knowledge in new methods and embrace improvements in predictive analytics. Ikechi works throughout all Mindshare accounts to make sure that analytics is constantly including worth via stakeholder partnership and clear storytelling.
Ikechi’s contributions to the trade have been highlighted in 2020 when he was chosen by Adweek as a Media All-Star for main the creation of Mindshare’s analytics and situation planning platform known as Synapse. Ikechi additionally takes time to attend and converse at varied conferences to remain linked with the analytics and advertising and marketing group. He’s an adjunct professor at Fordham and Tempo College and has sturdy relationships with different schools within the NY space (Columbia, Baruch, Simon Enterprise Faculty, and so on.) via organizing case competitions to supply experiential studying alternatives for the following era of analytics and advertising and marketing professionals.
[ad_2]