
[ad_1]
Abstract: A brand new examine highlights the challenges and developments in detecting AI-generated movies. Researchers have discovered that conventional digital media detection strategies falter towards movies produced by AI, corresponding to these created by the Sora generator from OpenAI.
By using a machine-learning algorithm, the crew efficiently recognized distinctive digital “fingerprints” left by varied AI video turbines. This growth is essential as AI-generated content material might doubtlessly be used for misinformation, necessitating strong detection methods to uphold media integrity.
Key Information:
- Conventional artificial picture detectors battle with AI-generated movies, with effectiveness dropping considerably in comparison with manipulated photos.
- Drexel’s crew has developed a machine studying method that may adapt to acknowledge the digital traces of various AI video turbines, even these not but publicly obtainable.
- The machine studying mannequin, after minimal publicity to a brand new AI generator, can obtain as much as 98% accuracy in figuring out artificial movies.
Supply: Drexel College
In February, OpenAI launched movies created by its generative synthetic intelligence program Sora. The strikingly sensible content material, produced through easy textual content prompts, is the most recent breakthrough for corporations demonstrating the capabilities of AI know-how.
It additionally raised considerations about generative AI’s potential to allow the creation of deceptive and deceiving content material on a large scale.
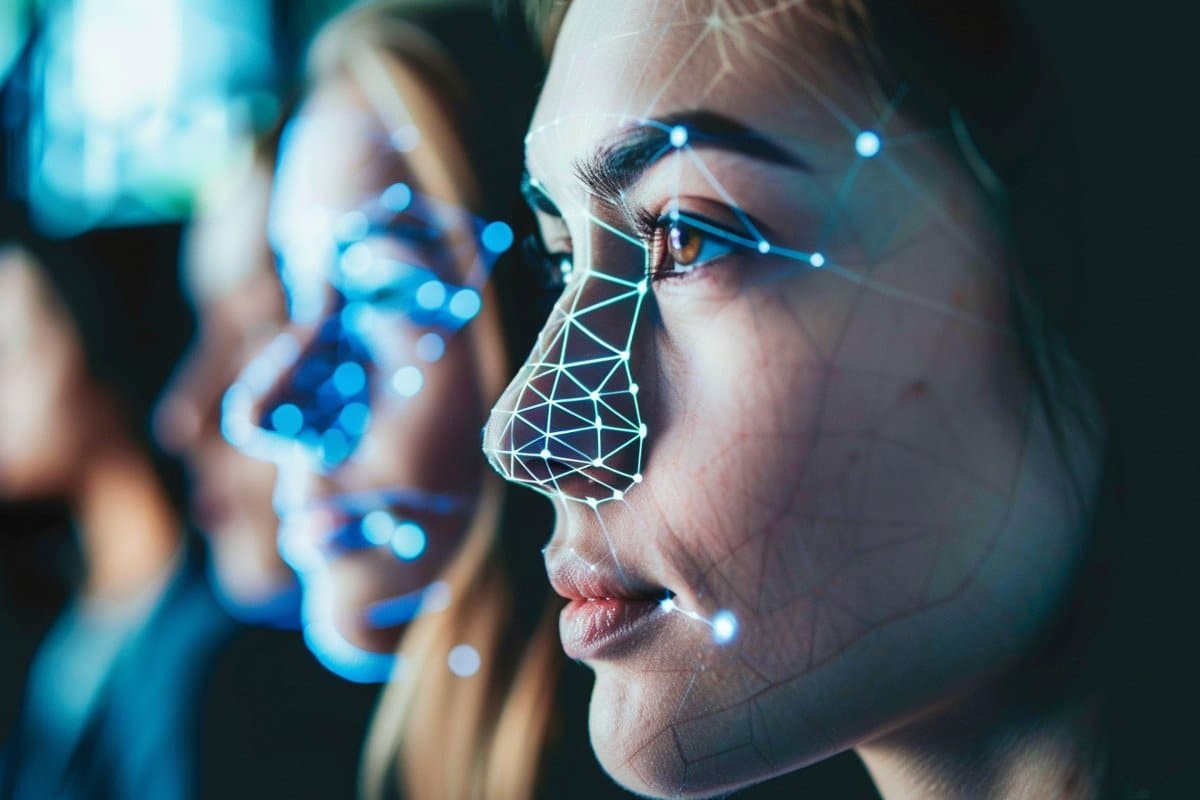
Based on new analysis from Drexel College, present strategies for detecting manipulated digital media is not going to be efficient towards AI-generated video; however a machine-learning method may very well be the important thing to unmasking these artificial creations.
In a paper accepted for presentation on the IEEE Laptop Imaginative and prescient and Sample Recognition Convention in June, researchers from Multimedia and Data Safety Lab in Drexel’s School of Engineering defined that whereas current artificial picture detection know-how has failed so far at recognizing AI-generated video, they’ve had success with a machine studying algorithm that may be skilled to extract and acknowledge digital “fingerprints” of many alternative video turbines, corresponding to Steady Video Diffusion, Video-Crafter and Cog-Video.
Moreover, they’ve proven that this algorithm can be taught to detect new AI turbines after learning only a few examples of their movies.
“It’s greater than a bit unnerving that this video know-how may very well be launched earlier than there’s a good system for detecting fakes created by unhealthy actors,” stated Matthew Stamm, PhD, an affiliate professor in Drexel’s School of Engineering and director of the MISL.
“Accountable corporations will do their finest to embed identifiers and watermarks, however as soon as the know-how is publicly obtainable, individuals who wish to use it for deception will discover a method. That’s why we’re working to remain forward of them by growing the know-how to establish artificial movies from patterns and traits which might be endemic to the media.”
Deepfake Detectives
Stamm’s lab has been energetic in efforts to flag digitally manipulated photos and movies for greater than a decade, however the group has been notably busy within the final 12 months, as enhancing know-how is getting used to unfold political misinformation.
Till just lately, these manipulations have been the product of photograph and video enhancing applications that add, take away or shift pixels; or sluggish, pace up or clip out video frames. Every of those edits leaves a singular digital breadcrumb path and Stamm’s lab has developed a collection of instruments calibrated to seek out and observe them.
The lab’s instruments use a classy machine studying program known as a constrained neural community. This algorithm can be taught, in methods just like the human mind, what’s “regular” and what’s “uncommon” on the sub-pixel stage of photos and movies, relatively than trying to find particular predetermined identifiers of manipulation from the outset.
This makes this system adept at each figuring out deepfakes from recognized sources, in addition to recognizing these created by a beforehand unknown program.
The neural community is often skilled on a whole bunch or 1000’s of examples to get an excellent really feel for the distinction between unedited media and one thing that has been manipulated — this may be something from variation between adjoining pixels, to the order of spacing of frames in a video, to the measurement and compression of the recordsdata themselves.
A New Problem
“Once you make a picture, the bodily and algorithmic processing in your digicam introduces relationships between varied pixel values which might be very completely different than the pixel values if you happen to photoshop or AI-generate a picture,” Stamm stated.
“However just lately we’ve seen text-to video turbines, like Sora, that may make some fairly spectacular movies. And people pose a very new problem as a result of they haven’t been produced by a digicam or photoshopped.”
Final 12 months a marketing campaign advert circulating in help of Florida Gov. Ron DeSantis appeared to indicate former President Donald Trump embracing and kissing Antony Fauci was the primary to make use of generative-AI know-how.
This implies the video was not edited or spliced collectively from others, relatively it was created whole-cloth by an AI program.
And if there is no such thing as a enhancing, Stamm notes, then the usual clues don’t exist — which poses a singular drawback for detection.
“Till now, forensic detection applications have been efficient towards edited movies by merely treating them as a sequence of photos and making use of the identical detection course of,” Stamm stated.
“However with AI-generated video, there is no such thing as a proof of picture manipulation frame-to-frame, so for a detection program to be efficient it can want to have the ability to establish new traces left behind by the way in which generative-AI applications assemble their movies.”
Within the examine, the crew examined 11 publicly obtainable artificial picture detectors. Every of those applications was extremely efficient — no less than 90% accuracy — at figuring out manipulated photos. However their efficiency dropped by 20-30% when confronted with discerning movies created by publicly obtainable AI-generators, Luma, VideoCrafter-v1, CogVideo and Steady Diffusion Video.
“These outcomes clearly present that artificial picture detectors expertise substantial issue detecting artificial movies,” they wrote. “This discovering holds constant throughout a number of completely different detector architectures, in addition to when detectors are pretrained by others or retrained utilizing our dataset.”
A Trusted Strategy
The crew speculated that convolutional neural network-based detectors, like its MISLnet algorithm, may very well be profitable towards artificial video as a result of this system is designed to continually shift its studying because it encounters new examples. By doing this, it’s doable to acknowledge new forensic traces as they evolve.
During the last a number of years, the crew has demonstrated MISLnet’s acuity at recognizing photos that had been manipulated utilizing new enhancing applications, together with AI instruments — so testing it towards artificial video was a pure step.
“We’ve used CNN algorithms to detect manipulated photos and video and audio deepfakes with dependable success,” stated Tai D. Nguyen, a doctoral pupil in MISL, who was a coauthor of the paper.
“As a result of their potential to adapt with small quantities of latest data we thought they may very well be an efficient answer for figuring out AI-generated artificial movies as properly.”
For the take a look at, the group skilled eight CNN detectors, together with MISLnet, with the identical take a look at dataset used to coach the picture detectors, which together with actual movies and AI-generated movies produced by the 4 publicly obtainable applications.
Then they examined this system towards a set of movies that included a quantity created by generative AI applications that aren’t but publicly obtainable: Sora, Pika and VideoCrafter-v2.
By analyzing a small portion — a patch — from a single body from every video, the CNN detectors have been in a position to be taught what an artificial video seems like at a granular stage and apply that information to the brand new set of movies. Every program was greater than 93% efficient at establish the artificial movies, with MISLnet performing one of the best, at 98.3%.
The applications have been barely simpler when conducting an evaluation of all the video, by pulling out a random sampling of some dozen patches from varied frames of the video and utilizing these as a mini coaching set to be taught the traits of the brand new video. Utilizing a set of 80 patches, the applications have been between 95-98% correct.
With a little bit of further coaching, the applications have been additionally greater than 90% correct at figuring out this system that was used to create the movies, which the crew suggests is due to the distinctive, proprietary method every program makes use of to provide a video.
“Movies are generated utilizing all kinds of methods and generator architectures,” the researchers wrote. “Since every method imparts important traces, this makes it a lot simpler for networks to precisely discriminate between every generator.”
A Fast Examine
Whereas the applications struggled when confronted with the problem of detecting a very new generator with out beforehand being uncovered to no less than a small quantity of video from it, with a small quantity of wonderful tuning MISLnet might rapidly be taught to make the identification at 98% accuracy.
This technique, known as “few-shot studying” is a crucial functionality as a result of new AI know-how is being created every single day, so detection applications have to be agile sufficient to adapt with minimal coaching.
“We’ve already seen AI-generated video getting used to create misinformation,” Stamm stated.
“As these applications turn into extra ubiquitous and simpler to make use of, we are able to fairly count on to be inundated with artificial movies. Whereas detection applications shouldn’t be the one line of protection towards misinformation — data literacy efforts are key — having the technological potential to confirm the authenticity of digital media is definitely an necessary step.”
About this AI and deepfake detection analysis information
Creator: Britt Faulstick
Supply: Drexel College
Contact: Britt Faulstick – Drexel College
Picture: The picture is credited to Neuroscience Information
Authentic Analysis: The findings shall be offered on the IEEE Laptop Imaginative and prescient and Sample Recognition Convention
[ad_2]