
[ad_1]
Abstract: Researchers have developed an AI methodology that may predict Alzheimer’s Illness as much as seven years earlier than the onset of signs, using machine studying to research affected person information.
Their examine highlights excessive ldl cholesterol and osteoporosis—notably in girls—as key predictors, showcasing AI’s potential to unveil complicated illness patterns and organic drivers. By integrating scientific knowledge with genetic databases by way of instruments like UCSF’s SPOKE, the group has recognized genes linked to Alzheimer’s, providing new avenues for early analysis and understanding the interaction between completely different well being situations and Alzheimer’s threat.
This strategy guarantees to boost precision drugs for Alzheimer’s and different difficult ailments.
Key Information:
- Early Prediction by way of AI: Machine studying utilized to scientific knowledge can predict Alzheimer’s onset with 72% accuracy as much as seven years upfront.
- Vital Predictors Recognized: Excessive ldl cholesterol and osteoporosis are important predictors of Alzheimer’s, with osteoporosis being a notable issue for ladies.
- Genetic Insights Unveiled: Utilizing UCSF’s SPOKE, researchers related Alzheimer’s threat to particular genes, together with a hyperlink between osteoporosis and Alzheimer’s in girls by way of the MS4A6A gene.
Supply: UCSF
UC San Francisco scientists have discovered a approach to predict Alzheimer’s Illness as much as seven years earlier than signs seem by analyzing affected person information with machine studying.
The situations that the majority influenced prediction of Alzheimer’s have been excessive ldl cholesterol and, for ladies, the bone-weakening illness osteoporosis.
The work demonstrates the promise of utilizing synthetic intelligence (AI) to identify patterns in scientific knowledge that may then be used to scour giant genetic databases to find out what’s driving that threat. The researchers hope that in the future it’s going to hasten the analysis and remedy of Alzheimer’s and different complicated ailments.
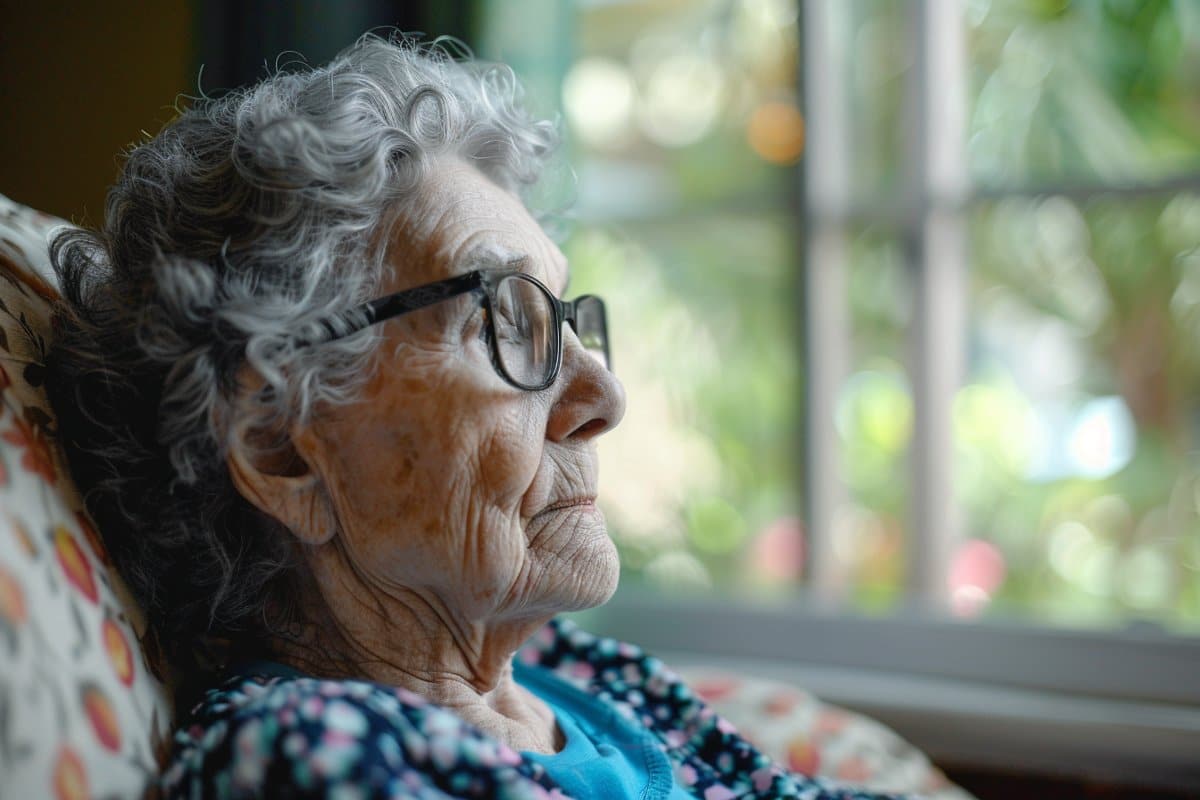
“This can be a first step in the direction of utilizing AI on routine scientific knowledge, not solely to determine threat as early as doable, but in addition to grasp the biology behind it,” mentioned the examine’s lead writer, Alice Tang, an MD/PhD pupil within the Sirota Lab at UCSF.
“The ability of this AI strategy comes from figuring out threat based mostly on combos of ailments.”
The findings seem Feb. 21, 2024, in Nature Growing older.
Medical knowledge and the ability of prediction
Scientists have lengthy sought to find the organic drivers and early predictors of Alzheimer’s Illness, a progressive and in the end deadly type of dementia that destroys reminiscence. Alzheimer’s impacts some 6.7 million Individuals, practically two-thirds of whom are girls. The chance of getting the illness will increase with age, and girls are likely to stay longer than males, however that doesn’t totally clarify why extra girls than males have it.
The researchers used UCSF’s scientific database of greater than 5 million sufferers to search for co-occurring situations in sufferers who had been recognized with Alzheimer’s at UCSF’s Reminiscence and Growing older Middle compared to people with out AD and located they might determine with 72% predictive energy who would develop the illness as much as seven years prior.
A number of components, together with hypertension, excessive ldl cholesterol and vitamin D deficiency, have been predictive in each women and men. Erectile dysfunction and an enlarged prostate have been additionally predictive for males. However for ladies, osteoporosis was a very essential predictor.
This doesn’t imply that everybody with the bone illness, which is widespread amongst older girls, will get Alzheimer’s.
“It’s the mixture of ailments that enables our mannequin to foretell AD onset,” mentioned Tang, “Our discovering that osteoporosis is one predictive issue for females highlights the organic interaction between bone well being and dementia threat.”
A precision drugs strategy
To grasp the biology underlying the mannequin’s predictive energy, the researchers turned to public molecular databases and a specialised software developed at UCSF referred to as SPOKE (Scalable Precision Medication Oriented Data Engine), which was developed within the lab of Sergio Baranzini, PhD, a professor of neurology and a member of the UCSF Weill Institute for Neurosciences.
SPOKE is basically a database of databases that researchers can use to determine patterns and potential molecular targets for remedy. It picked up the well-known affiliation between Alzheimer’s and excessive ldl cholesterol, by way of a variant type of the apolipoprotein E gene, APOE4. However, when mixed with genetic databases, it additionally recognized a hyperlink between osteoporosis and Alzheimer’s in girls, by way of a variant in a lesser-known gene, referred to as MS4A6A.
In the end, the researchers hope the strategy can be utilized with different hard-to-diagnose ailments like lupus and endometriosis.
“This can be a nice instance of how we will leverage affected person knowledge with machine studying to foretell which sufferers usually tend to develop Alzheimer’s, and likewise to grasp the the explanation why that’s so,” mentioned the examine’s senior writer, Marina Sirota, PhD, affiliate professor on the Bakar Computational Well being Sciences Institute at UCSF.
Authors: Extra UCSF co-authors embody Katherine P. Rankin, PhD, Gabriel Cerono, MD, Silvia Miramontes, MIDS, Hunter Mills, MS, Jacquelyn Roger, PhD pupil, Billy Zeng, MD, Charlotte Nelson, PhD, Karthik Soman, PhD, Sarah Woldemariam, Yaqio Li, PhD, Albert Lee, MADS, Riley Bove, MD, Tomiko Oskotsky, MD, Zachary Miller, MD, Isabel Allen, PhD, Stephan J. Sanders, PhD, and Sergio Baranzini, PhD.
Funding: The Nationwide Institute on Growing older supplied the first help for this examine (grant R01AG060393). Extra help was supplied by the Medical Scientist Coaching Program (T32GM007618) and F30 Fellowship (1F30AG079504-01).
About this AI and Alzheimer’s illness analysis information
Writer: Victoria Colliver
Supply: UCSF
Contact: Victoria Colliver – UCSF
Picture: The picture is credited to Neuroscience Information
Unique Analysis: Open entry.
“Leveraging digital well being information and data networks for Alzheimer’s illness prediction and sex-specific organic insights” by Alice Tang et al. Nature Growing older
Summary
Leveraging digital well being information and data networks for Alzheimer’s illness prediction and sex-specific organic insights
Identification of Alzheimer’s illness (AD) onset threat can facilitate interventions earlier than irreversible illness development. We exhibit that digital well being information from the College of California, San Francisco, adopted by data networks (for instance, SPOKE) enable for (1) prediction of AD onset and (2) prioritization of organic hypotheses, and (3) contextualization of intercourse dimorphism.
We educated random forest fashions and predicted AD onset on a cohort of 749 people with AD and 250,545 controls with a imply space below the receiver working attribute of 0.72 (7 years prior) to 0.81 (1 day prior). We additional harnessed matched cohort fashions to determine situations with predictive energy earlier than AD onset.
Data networks spotlight shared genes between a number of prime predictors and AD (for instance, APOE, ACTB, IL6 and INS). Genetic colocalization evaluation helps AD affiliation with hyperlipidemia on the APOE locus, in addition to a stronger feminine AD affiliation with osteoporosis at a locus close to MS4A6A.
We due to this fact present how scientific knowledge might be utilized for early AD prediction and identification of customized organic hypotheses.
[ad_2]